The benefits of using AI to enhance efficiency, drive innovation, and unlocking new opportunities have been well documented.
However, as organisations in APAC start to plan for AI, they are learning that they need to navigate the complexities and potential pitfalls that come with implementing AI’s transformative technologies.
Many face challenges such as a lack of expertise, integration difficulties as well as security and data infrastructure or management concerns. The lack of a proper framework and strategy can lead to inefficient deployments or wasted investments, and cause companies to miss out on AI’s full potential.
How should we prepare to adopt AI, in a way that can deliver significant and sustainable value for the business?
In our special focus on AI Adoption for this month, iTNews Asia speaks to industry practitioners on what will make a well-defined strategy and roadmap for AI adoption, while ensuring sustainable growth and success. We also sought recommendations on how they can align their business goals, mitigate risks, and address ethical concerns from AI.
Our respondents include:
Donald MacDonald, Head of Group Data Office, OCBC;
Chris Lewin, AI and Data Capability Leader, Asia Pacific, Deloitte;
Santh Raul, Assistant General Manager, Aditya Birla Management;
Pravin G Neel, Chief Operating Officer & Director, DiaSys Diagnostics India; and
Benjamin Chan, Research Analyst, ABI Research
iTNews Asia: What are the most common challenges organisations face when adopting AI technologies? Can you share examples of successful AI applications?
MacDonald (OCBC): Other than technical considerations, such as the availability of quality data, infrastructure and computing power, integration capabilities, and use case identification, the buy-in from management is crucial to ensure that AI is being applied to the opportunities that really matter to the business.
AI is utilised throughout OCBC to improve customer experience, mitigate risks, and enhance productivity. Its applications span customer service, personalisation, fraud detection and prevention, risk management, process automation, and data analytics.
Generative AI (GenAI), has been a game changer for us. The use of GenAI has been focused on employee productivity. We have an arsenal of in-house tools to bolster the employee experience, such as our “Buddy” Knowledge Assistant to quickly find pertinent company information, a document summarisation tool, a coding co-pilot for developers, and an instant speech-to-text tool for contact centre agents at our call centre.
Raul (Aditya Birla): Data quality and availability pose significant challenges in leveraging AI technologies for business decisions. Organisations often face fragmented or siloed data sources, complicating the creation of a comprehensive knowledge base for AI. Additionally, maintaining data accuracy, reliability, currency, and neutrality is difficult.
Talent and expertise are also critical, as developing, deploying, and maintaining AI solutions requires specialised skills in machine learning, data engineering, and model interpretability. A shortage of skilled personnel can impede progress and complicate troubleshooting. Organisations need to restructure in alignment with their business operations, rather than in isolation.
Finally, change management is essential, as people are key to the success of AI technology. Clearly identifying the right problems to solve and involving business teams from the outset of solution development can enhance adoption rates.
Successful AI applications implemented within our firm include early asset fault detection in manufacturing, supply chain optimisation, and customer sentiment analysis.
Chan (ABI): First, it’s essential to differentiate between internal and external AI applications. Internal AI refers to applications developed for specific enterprise use, either self-created or based on third-party Large Language Models (LLMs), while external AI encompasses public tools like ChatGPT or Bard that aid daily operations.
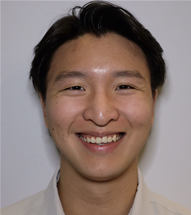
Effective AI applications rely heavily on data fidelity and comprehensiveness, yet many enterprises still depend on legacy IT systems that only support basic data collection and processing. This often results in unstructured data that requires significant cleansing.
– Benjamin Chan, Research Analyst, ABI Research
Further, legacy infrastructure may suffice for everyday operations, companies are often hesitant to invest in new technology and AI teams, especially when short-term ROI from AI implementations isn’t immediately apparent.
Similar to the introduction of other new technologies, there is usually considerable resistance to changes in workplace processes, compounded by a lack of skills and knowledge among the workforce about using AI tools effectively, which necessitates additional training investments.
Neel (DiaSys): The challenges include a lack of quality data, concerns over data authenticity, the need for thorough validation and verification, and a lack of confidence among management and leadership in AI expertise and its effective use.
We have notable applications of AI, such as Google Maps, data predictions during the COVID-19 pandemic, and various e-commerce solutions, demonstrating the potential of leveraging data for improved outcomes.
Lewin (Deloitte): Organisations are now facing challenges in scaling AI effectively. AI initiatives sometimes remain siloed within specific functions, limiting their impact.
Overcoming these barriers requires coordinated leadership, building ‘trustworthy AI’, as well as robust data infrastructure and technology to enable AI to scale cost-effectively. Scaling AI also requires a fit-for-purpose operating model, with an emphasis on both providing business value and enabling adoption.
iTNews Asia: What key indicators should companies evaluate to assess their AI readiness? What should be the essential considerations when preparing their AI roadmap? What will be a reasonable timeline for the AI to see ROI results?
Chan (ABI): Evaluating AI readiness should be done in stages, focusing on key parameters like organisation, infrastructure, data, and business value to ensure a thorough assessment for AI adoption.
- Organisation: Key questions include whether there is a clear vision for AI implementation, an established governance framework for data handling, and a strategy for building AI capabilities through talent recruitment and training.
- Business Value: Organisations should identify pain points or processes that AI can improve and consider how AI can provide a competitive advantage.
- Data: It’s essential to determine the data needed to create business value and to develop a standardised data format across the organisation.
- Infrastructure: Organisations must identify the systems within their network and decide if AI processing should occur on enterprise servers or in the cloud.
Raul (Aditya Birla): To evaluate AI readiness, companies should focus on key indicators across data, technology, skills, and organisational alignment as well as budget and resources.
When assessing ROI, it’s important to break it down into stages. An AI strategy must align with business strategy, starting with specific goals related to customer experience, efficiency, cost reduction, or innovation that reflect broader business priorities.
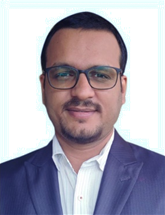
Early pilots in areas with ample data and clear objectives – like customer service chatbots or marketing optimisation – can yield measurable benefits within the first year, often resulting in incremental ROI from improved efficiency or minor cost savings.
– Santh Raul, Assistant General Manager, Aditya Birla Management;
More complex applications, such as predictive maintenance or advanced customer segmentation, typically show ROI over one to two years, allowing time for refined model training, implementation, change management, and impact measurement.
For strategic, enterprise-wide AI applications – like supply chain optimisation or end-to-end process automation – ROI expectations extend to three or more years, as these initiatives require significant data gathering, interdepartmental collaboration, and real-world performance adjustments.
Ultimately, AI readiness depends on robust data foundations, adequate technical and human resources, and organisational commitment. Developing a roadmap with clear objectives, piloting projects, and planning for ongoing improvement is vital.
While basic AI applications may generate ROI within a year, more complex implementations usually take 1-3 years to fully realise their return, based on their scope and alignment with the organisation.
MacDonald (OCBC): For a company to scale effectively and reap the benefits of AI technologies, our goal is to ensure AI is embedded deeply into the processes and systems used by our employees and customers every day. AI cannot scale if it’s seen as a standalone application separate from the way that people work.
Given that it takes time to scale, being an early adopter helps. One of the reasons I think OCBC has moved so fast on GenAI is that we were already using the first generation of large language models back in 2019 and 2020. When OpenAI’s large language model GPT-3 and ChatGPT took off at the end of 2022, we were able to move quickly to make our existing applications better.
Neel (DiaSys): Key indicators for AI readiness include data quality, data infrastructure, the technical skill sets of those using the data, and adherence to risk management and ethical practices. An effective AI roadmap should articulate a clear vision, mission, and goals for implementation at the organisational level, ensuring that this information is communicated to all stakeholders.
Regarding ROI, a reasonable timeline is typically three to four years, provided the data is reliable and well-structured, and the IT team is proficient in various tools and techniques.
Lewin (Deloitte): There are several indicators for AI readiness including commitment from top management to drive AI at scale. It is a ‘team sport’ that needs different parts of the organisation to work together.
Organisations should work on a roadmap for technology and data infrastructure that is capable of supporting different AI workloads across the organisation.
iTNews Asia: What necessary steps can companies take to ensure their IT infrastructure can support AI? What are the lessons you’ve learnt or mistakes we can avoid?
MacDonald (OCBC): OCBC has been modernising its architecture over the last five years with a focus on containerisation, DevOps and microservices – as well as our own purpose-built data centre. These capabilities have helped accelerate our AI journey as the data teams were able to build our MLOps (machine learning operations) processes on top of IT processes. This enabled us to automate our model deployment and monitoring processes, allowing us to get to market quickly. Having our own data centre also allowed us to build our own internal GPU clusters.
This was a key unlock when deploying our GenAI models as we were able to quickly build applications using on-premise open-source Large Language Models without having to worry so much about external data leakage or cost challenges that might have arisen if we were using external API-based models.
Raul (Aditya Birla): To ensure IT infrastructure can support AI effectively, organisations should consider a multi-step approach, focusing on robust data handling, high-performance computing resources, and scalability.
Up to proof of concept (POC) stage, the point solution and disintegrated data infrastructure is fine. But when going for the pilot stage, the underlying robust data infrastructure should be prepared.
Avoid common mistakes such as neglecting MLOps, underestimating the problem discovery and aligning required data to solve the problem, focusing too narrowly on short-term cost savings etc. By planning with these considerations in mind, companies can set up a resilient, scalable infrastructure to support successful AI initiatives.
Neel (DiaSys): Start by studying your current processes to define a value stream map for future improvements. It’s crucial to have a Chief Transformation Officer (CTO) who can champion the organisation’s vision, mission, and goals, focusing on the 6Ms: Man, Machine, Method, Measurement, Mother Nature, and Material.
iTNews Asia: What are your observations of mistakes companies can avoid when looking at AI, or lessons learnt from early adopters?
Lewin (Deloitte):
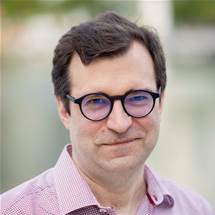
A critical lesson from early AI adopters is the importance of starting with the end in mind. By being specific about the business objectives such as improving customer experience, streamlining operations, or driving innovation – organisations can better ‘hold the course’ towards ultimately delivering value, and driving impact.
– Chris Lewin, AI and Data Capability Leader, Asia Pacific, Deloitte
AI in enterprises is not a ‘magic box’, and so having a clear direction consistently helps as decisions need to be made and re-made, or engineering approaches need to be adjusted along the way.
Chan (ABI): ABI Research projects that revenue from Generative AI software will hit US$176 billion by 2030, growing at a CAGR of 50 percent. For companies looking to quickly gain value as early adopters, the key to achieving AI-enabled ROI lies in effective use of data and data processes. These include:
- Clean Data: Insights should come from a single, accurate source of truth. Poor data quality like duplicates or missing entries can distort analysis. A strong data governance strategy is essential.
- Relevant Data: Using up-to-date and relevant data improves the reliability of AI models and maximises ROI. Outdated or incomplete data can lead to misleading results.
- Proprietary Data: Companies need proper AI governance to protect sensitive consumer data from being inadvertently shared online, which could undermine their competitive edge.
- Purposeful AI Adoption: Organisations should implement AI with a clear understanding of how it will create business value, rather than just to keep up with tech trends.
iTNews Asia: What practices can be followed for maintaining legacy systems while adopting AI?
Lewin (Deloitte): Companies with legacy systems can adopt a hybrid approach that combines legacy systems with modern AI technologies. By assessing the current state, they can identify components of legacy systems that can be enhanced with AI without full replacement.
Then gradually, they can upgrade system components while maintaining core functionalities. Middleware solutions will also help to bridge the gap between legacy systems and new AI applications. At the same time, companies can get their employees managing legacy systems to upskill on AI technologies to stay relevant and add more value to the business.
Neel (DiaSys):
To successfully integrate AI with legacy systems, it’s crucial to focus on meticulous data quality management and ongoing modernisation through refactoring and data integration.
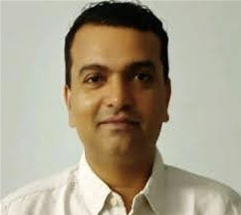
Companies should evaluate their modernisation strategies carefully and manage change thoughtfully to ensure smooth data extraction from legacy systems. Implementing AI as an additional layer can enhance existing functionalities gradually.
– Pravin G Neel, Chief Operating Officer & Director, DiaSys Diagnostics India
Prioritising data quality is essential for training accurate AI models, and continuous monitoring and adaptation of AI systems are necessary to ensure they align well with legacy operations.
Raul (Aditya Birla): Maintaining legacy systems while adopting AI requires a careful balance to introduce new AI-driven capabilities without disrupting essential operations. Begin by integrating AI in specific, isolated areas through APIs to enhance features without overhauling the entire system. Implement a data pipeline that extracts data from legacy systems, transforms it into a usable format, and feeds it into AI models.
If legacy systems generate a large amount of data, consider using a data lake to store and pre-process this information, allowing AI models to access it from there instead of directly from the legacy system.
Middleware and edge solutions can bridge the gap between legacy systems and modern AI platforms by translating formats and protocols to ensure compatibility, minimising direct changes to the legacy systems. Instead of replacing legacy systems all at once, develop a phased roadmap to gradually replace or modernise components, ensuring business continuity.
A hybrid approach, combined with monitoring, MLOps, and security practices, will help maintain stability in legacy systems as AI capabilities are integrated. This strategy allows companies to leverage AI advancements while keeping their legacy systems reliable.
Chan (ABI): Companies need to evaluate the capital (CAPEX) and operational (OPEX) investments required to adopt and operate the identified AI systems alongside their existing infrastructure.
Moreover, there are emerging solutions in the market that assist enterprises in integrating AI/ML applications into their legacy systems.
Since legacy systems often produce unstructured data, AI-based enterprise planning systems can be valuable for managing, cleaning, and transforming this data into actionable insights, such as predictive analytics and real-time tasks.
MacDonald (OCBC): Instead of a complete overhaul, perhaps consider a modular approach where updates or replacements of legacy systems can be done gradually. At the same time, we also deploy our models as microservice endpoints and even legacy systems can integrate with these to get value from the AI models.
iTNews Asia: What ethical considerations and governance frameworks should companies take to ensure responsible AI use?
Lewin (Deloitte): At Deloitte, we have developed a trustworthy AI framework to help design and establish a sustainable, safe, and responsible environment for AI. It considers various dimensions to manage the exposures and risks related to the introduction of AI systems.
To address fairness and bias, organisations should audit models, use diverse datasets, and implement fairness metrics. For transparency and explainability, developing explainable AI (XAI) models and documenting decision processes is essential. Accountability can be ensured by defining roles, implementing reporting mechanisms, and maintaining human oversight.
Regarding privacy and security, adherence to regulations, strong cybersecurity measures, and data anonymisation are crucial. Developing an ethics code and incorporating ethics throughout all phases of AI development, along with regular reviews, helps foster ethical AI practices. Finally, a human-centric approach should focus on augmenting human capabilities, avoiding job displacement, and providing necessary training.
MacDonald (OCBC): In Singapore, the financial regulator (MAS) worked in partnership with the banks to define the FEAT principles for responsible use of AI.
OCBC has operationalised these principles within our model management platform to ensure that model use is Fair, Ethical, Accountable and Transparent and we continue to work with the regulators on the next generation of such controls.
For example, we have deployed tools to help us identify and reduce potential “hallucinations” from our GenAI applications so we can be confident that answers provided to users are accurate.
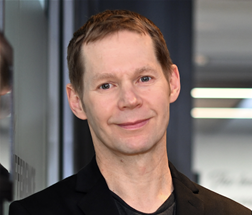
As we are heavy users of open-source LLMs, we have built an arsenal of tools to automatically test the new LLMs upon release to understand where the models are strong and where they may have issues with bias or inequality. We have also embedded fairness testing into our machine learning platforms to ensure that all models are automatically assessed for fairness prior to use.
– Donald MacDonald, Head of Group Data Office, OCBC
Raul (Aditya Birla): A strong governance framework for responsible AI involves transparent operations, data privacy, data ethics, continuous monitoring, employees and community considerations etc. By establishing these ethical standards and governance structures, we usually maximise the benefits of AI while upholding accountability and social responsibility.
Chan (ABI): Companies must form comprehensive AI and data governance measures to ensure responsible AI implementation. Data governance is the most critical issue, as concerns regarding the use of internal data for training models can be mitigated with good governance practices.
Some ethical considerations to be noted include data breach, privacy protection, biases and discrimination. To ensure this, robust data management and cleaning need to be done.
Neel (DiaSys): Ethical guidelines for AI developers should include transparency provisions, ensuring AI systems disclose their decision-making data sources and processes. Ethical considerations must extend to addressing biases in AI algorithms, emphasising fairness, and actively working to eliminate discriminatory outcomes.
iTNews Asia: What is your long-term vision for integrating AI into your operations?
MacDonald (OCBC): We launched our first GPT tool to all employees in 2023. Our approach is to empower every employee with GenAI universal assistants that help all OCBC employees be more efficient. We then go deep and build role-specific co-pilots that focus on empowering employees in functions such as IT, sales or service teams. These tools are more integrated into the process and platforms of each team, leading to greater productivity gains.
We believe our GenAI tools have the potential to transform the way our employees work by automating a wide range of time-consuming tasks, freeing up their time to focus on more strategic and value-added work.
Raul (Aditya Birla): Our long-term vision for integrating AI focuses on transforming business processes to boost productivity, sustainability, and strategic decision-making. We see AI not just as a technology but as a catalyst for operational excellence and innovation across our diverse segments.
We focus AI integration towards process automation, data-driven insights, and sustainability. We aim to automate routine and complex tasks alike, whether in manufacturing, supply chain logistics, or customer service.
Predictive analytics help us optimise production scheduling, improve demand forecasting etc. Our goal is to make smarter, data-backed decisions at every level, helping us stay agile and competitive in rapidly changing markets.
From energy optimisation in production to waste management, we believe AI will play a key role in achieving our sustainability goals, supporting both economic and environmental value.
iTNews Asia: What advice can you give to companies in APAC on how they can assess the long-term impact of AI adoption on their operations?
Lewin (Deloitte): There are a variety of metrics to track the progress of AI adoption, ideally with key performance indicators (KPIs) aligned to business objectives. Then the expectation of ROI results should correspond with the level of investment and adoption of AI over time.
The timeline for AI integration is divided into three phases. In the short term (6-12 months), organisations should look for early signs of success and quick wins, such as efficiency improvements, cost savings, and enhanced customer experiences.
In the medium term (12-24 months), the focus shifts to achieving substantial results through scaled AI, including increased revenue and better decision-making.
Finally, in the long term (24+ months), the goal is to fully realise AI’s potential with strategic integration, leading to competitive advantage, sustained growth, and operational excellence.
Chan (ABI): Different variations of AI implementations have differing timelines for ROI realisation. AI chatbots, like Mastercard’s AI integrated chatbots, and unstructured data handling systems generally see a shorter time to ROI.
Comparatively, larger autonomous AI projects like autonomous mobile robots or automation-based innovation like Honeywell’s AI-driven OT automation could take much longer.
Typically, some impact parameters that could be useful include operational savings – evaluating operational savings due to process automation and efficiency improvements; product innovation and market differentiation where many differentiation strategies can be gleaned from generative AI insights. Possible measures for this include product sale performance and brand loyalty or impression.