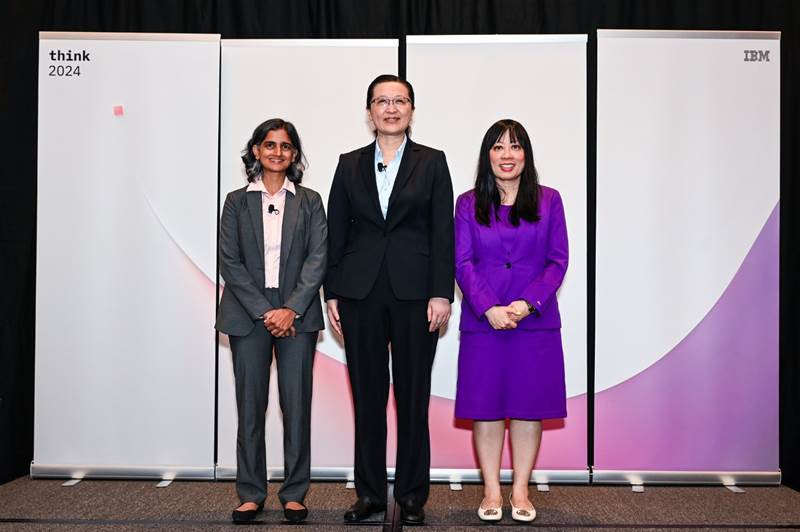
Priya Nagpurkar, Vice President, Hybrid Cloud and AI Platform, IBM Research; Prof Liu Bin, Deputy President (Research & Technology), NUS; Catherine Lian, General Manager and Technology Leader, IBM ASEAN
The National University of Singapore (NUS) is collaborating with IBM to build a new AI research and innovation centre. The centre, expected to be set up at NUS School of Computing in 2025, will feature the first such full-stack AI infrastructure system installed by IBM on a university campus in Asia-Pacific.
The collaboration follows a commitment made by the country’s research and innovation ecosystem to collaborate with industry leaders and tech companies, such as IBM, to realise Singapore’s National AI Strategy. The centre will aim to serve as an innovation platform for Singapore agencies, academic and research institutions, as well as companies to jointly conduct cutting-edge AI research with significant translational potential.
The AI-optimised computing infrastructure would operate on the IBM watsonx data and AI platform and Red Hat hybrid cloud platform. The collaboration also taps on NUS’ expertise to enable more powerful, efficient, and versatile AI systems that can tackle increasingly complex tasks.
The collaboration would enable the NUS Graduate Research Innovation Programme (GRIP), a deep tech entrepreneurship program, together with local startups as well as small and medium-sized enterprises, to gain access to the AI Innovation Platform.
Taking a sustainability and open innovation approach
A cornerstone of the centre will be to advance a sustainability focused open innovation approach to developing AI technologies across the stack and to develop tools and methodologies that help build trust in AI.
This includes doing research in Green AI and AI Safety. Under Green AI, the parties hope to look at developing AI technologies and methodologies that are energy-efficient and environmentally friendly. This could help reduce the carbon footprint and resource consumption associated with AI development and deployment.
Some possible examples include creating energy-efficient AI models; and optimising algorithms to run on smaller, less powerful devices without significant performance loss; and leveraging AI to address environmental challenges such as climate modelling and optimising energy grids.
Under AI Safety, the parties will look at how AI systems (such as AI models and software platforms) can be designed, developed, and deployed in ways that are safe, reliable, and achieves beneficial outcomes.
In this area, examples include enhancing the robustness of AI systems so that they can perform reliably; protecting AI systems from malicious attacks; and addressing and mitigating biases in AI systems.